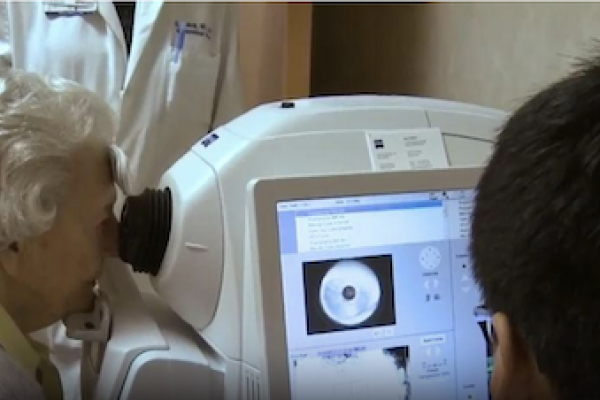
Advancements in Optical Coherence Tomography and Neurodegenerative Disease
Neurodegenerative diseases are becoming ever more prevalent in our aging population. Given the debilitating and progressive nature of these diseases, early and cost-effective diagnosis of these diseases is paramount. However, conventional diagnostics such as brain magnetic resonance imaging, positron emission tomography, cerebrospinal fluid biomarkers, and genetic testing are expensive and not often readily available, which implies a more accessible alternative. Retinal imaging may be a potential alternative given that neurodegenerative changes in the brain have been associated with structural and microvascular changes in the retina, and machine learning models have been developed to distinguish retinal images using optical coherence tomography (OCT) and OCT angiography (OCTA) of patients with cognitive impairment and Alzheimer’s disease from those of healthy controls.
Machine Learning Model Identifies Mild Cognitive Impairment from Retinal Scans
The tool demonstrates the potential for a non-invasive and inexpensive way to diagnose a common precursor of Alzheimer’s disease.
A machine learning model developed by Duke Health researchers can differentiate normal cognition from mild cognitive impairment using retinal images from the eye.
The model analyzes retinal images and associated data and recognizes specific features to identify individuals with mild cognitive impairment. Publishing in the journal Ophthalmology Science, the model demonstrates the potential for a non-invasive and inexpensive method of identifying the early signs of cognitive impairment that could progress to Alzheimer’s disease.
“This is particularly exciting work because we have previously been unable to differentiate mild cognitive impairment from normal cognition in previous models,” said senior author Sharon Fekrat, M.D., professor in Duke’s departments of Ophthalmology and Neurology, and associate professor in the Department of Surgery. “This work brings us one step closer to detecting cognitive impairment earlier before it progresses to Alzheimer’s dementia.”
Fekrat and colleagues previously developed a model that used retinal scans and other data to successfully identify patients with a known Alzheimer’s diagnosis. The scans – based on optical coherence tomography (OCT) and OCT angiography (OCTA) -- detected structural changes in the neurosensory retina and its microvasculature among Alzheimer’s patients.
The current study expands on that work, using machine learning techniques to detect mild cognitive impairment, which is often a precursor to Alzheimer’s. The new model identifies specific features in the OCT and OCTA images that signal the presence of cognitive impairment, along with patient data such as age, sex, visual acuity, and years of education and quantitative data from the images themselves.
The researchers reported that the model analyzed retinal pictures and images along with quantitative data to differentiate people with normal cognition from those with a diagnosis of mild cognitive impairment with a sensitivity of 79% and specificity of 83%.
“This is the first study to use retinal OCT and OCTA images to distinguish people with mild cognitive impairment from individuals with normal cognition,” said co-first author C. Ellis Wisely, M.D., assistant professor in the Department of Ophthalmology.
“Having a non-invasive and less expensive means to reliably identify these patients is increasingly important, particularly as new therapies for Alzheimer’s disease may become available,” Wisely said.
“The retina is a window to the brain, and machine learning algorithms that leverage non-invasive and cost-effective retinal imaging to assess neurological health can be a potent tool to screen patients at scale,” said co-lead author Alexander Richardson, a student in the Eye Multimodal Imaging in Neurodegenerative Disease lab at Duke.
In addition to Fekrat, Wisely and Richardson, study authors include Ricardo Henao, Cason B. Robbins, Justin P. Ma, Dong Wang, Kim G. Johnson, Andy J. Liu, and Dilraj S. Grewal.
OphthalmologyScience2024;4:100355
Convolutional Neural Network-based Automated Quality Assessment of OCT and OCT Angiography Image Maps in Individuals with Neurodegenerative Disease
Retinal imaging may be a potential alternative given that neurodegenerative changes in the brain have been associated with structural and microvascular changes in the retina, and machine learning models have been developed to distinguish retinal images (optical coherence tomography [OCT] and OCT angiography [OCTA] of patients with Alzheimer’s disease from those of healthy controls.
One critical shortcoming of machine learning to distinguish retinal images OCT and OCTA is the susceptibility images to artifacts that impact scan quality, more so in those with cognitive impairment. As a result, manual grading is required to determine the quality of each individual image, which is not only very time and resource intensive but also prone to human error. To more seamlessly use automated software, built-in automated image quality control would be paramount. In this study, published in Translational Vision Science and Technology, members of the iMind research team and collaborators, led by Terry Lee, MD developed a machine learning model to accurately classify the image quality of OCTA scans of the fovea as well as OCT color maps of ganglion cell-inner plexiform layer, demonstrating that machine learning models can be trained to accurately assess OCT and OCTA image quality. Since good-quality retinal images are critical for the accurate assessment of microvasculature and structure, incorporating an automated image quality sorter may obviate the need for manual image review and allow for the development of standalone software for the detection of neurodegenerative diseases using retinal imaging.
In addition to Lee, Alexandra Rivera, Matthew Brune, Anita Kundu, Alice Haystead, Lauren Winslow, Raj Kundu, C. Ellis Wisely, Cason Robbins, Ricardo Henao, Dilraj Grewal, and Sharon Fekrat.
Translational Vision Science & Technology June 2023, Vol.12, 30
Characterizing differences in retinal and choroidal microvasculature and structure in dementia with Lewy bodies compared to normal cognition
This research study, conducted by the iMIND study group, analyzes optical coherence tomography (OCT) and OCT angiography (OCTA) images in eyes of participants with Dementia with Lewy Bodies (DLB) compared to cognitively intact controls. We identified increased peripapillary capillary perfusion density, decreased peripapillary capillary flux index, and attenuated ganglion cell-inner plexiform layer thickness in individuals with DLB compared to controls.
These findings, published in the Journal of VitreoRetinal Diseases, first author Suzanna Joseph, BS, Duke 3rd Year Medical Student, suggests that retinal imaging metrics have the potential to serve as clinically relevant biomarkers for DLB diagnosis.
This is the first cross-sectional study to use OCTA to evaluate the peripapillary RPC plexus in patients with DLB. Because of the limited literature on retinal biomarkers in DLB, we believe our findings represent a meaningful contribution to the literature and can advance the current understanding of retinal and choroidal imaging biomarkers associated with DLB.
In addition Cason B Robbins, Ariana Allen, Alice Haystead, Angela Hemesath, Anita Kundu, Kimberly G. Johnson, Justin P. Ma, Rupesh Agrawal, Vithiya Gunasan, Sandra S. Stinnett, Dilraj S. Grewal, and Sharon Fekrat.
Journal of VitreoRetinal Diseases January 2024, Vol 8,1, Pages 67-74